Ensemble Methods
Machine Learning Ensemble Methods
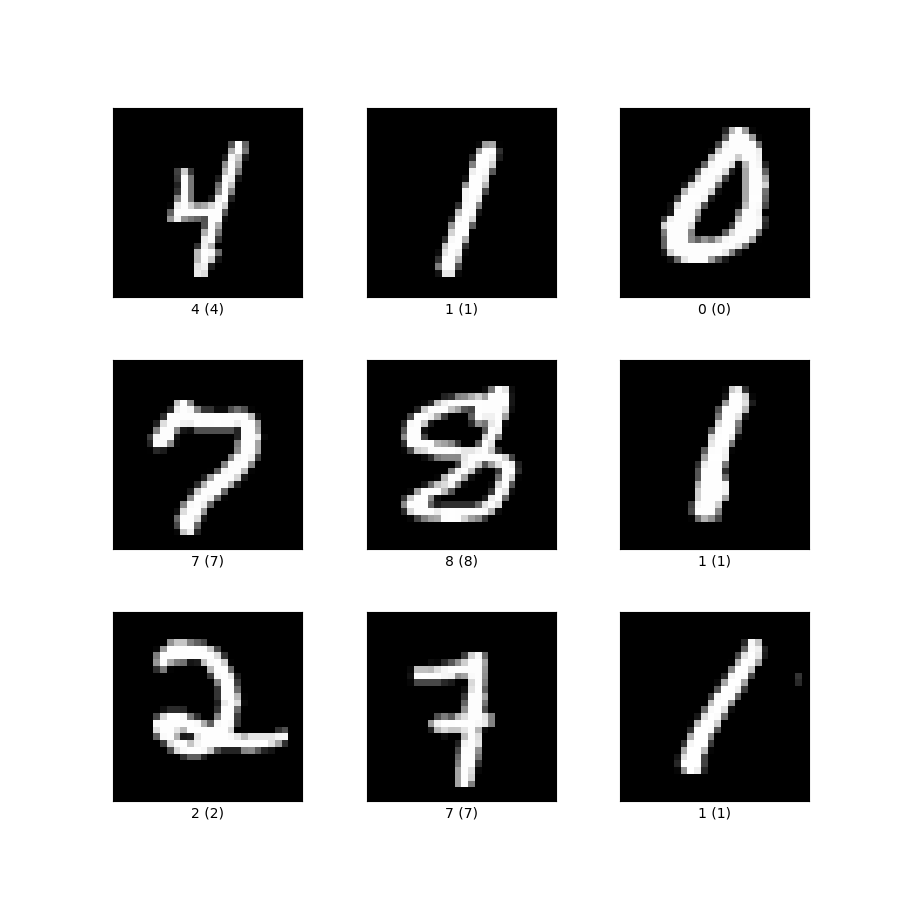
.
This project investigates the power of ensemble machine learning models for the MNIST dataset. The individual components are made up of
- Random Forests
- Extra Trees
- Support Vector Machines
Two varieties of ensembling are constructed. They are
Ensemble 1 - Soft Voting Classifier
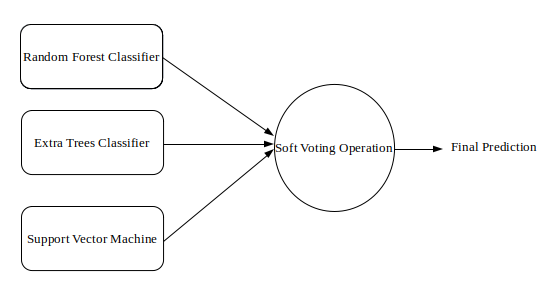
.
This combines the 3 classifier algorithms and performs a voting mechanism to determine the class that the image belongs to.
Ensemble 2 - Stacking Classifier
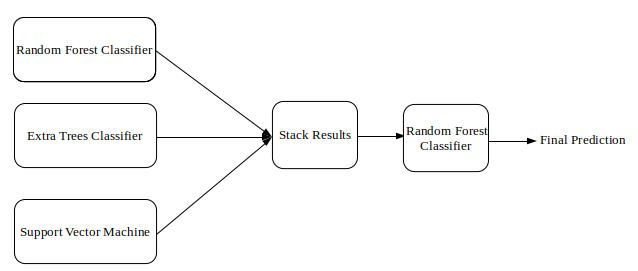
.
Stacking Classifier uses a two tier architecture. In the first stage, the output of three classifier are stacked and fed into a random forest classifier. This mimics a feature extraction stage followed by classifier, which is quite common in deep neural networks.
Results
Individual Models
Model | Test Set Accuracy (in percentage) |
---|---|
Random Forests | 96.87 |
Extra Forests | 97.07 |
Support Vector Machines | 96.46 |
Ensemble Models
Model | Test Set Accuracy (in percentage) |
---|---|
Soft Voting Classifier | 97.42 |
Stacking Classifier | 97.08 |